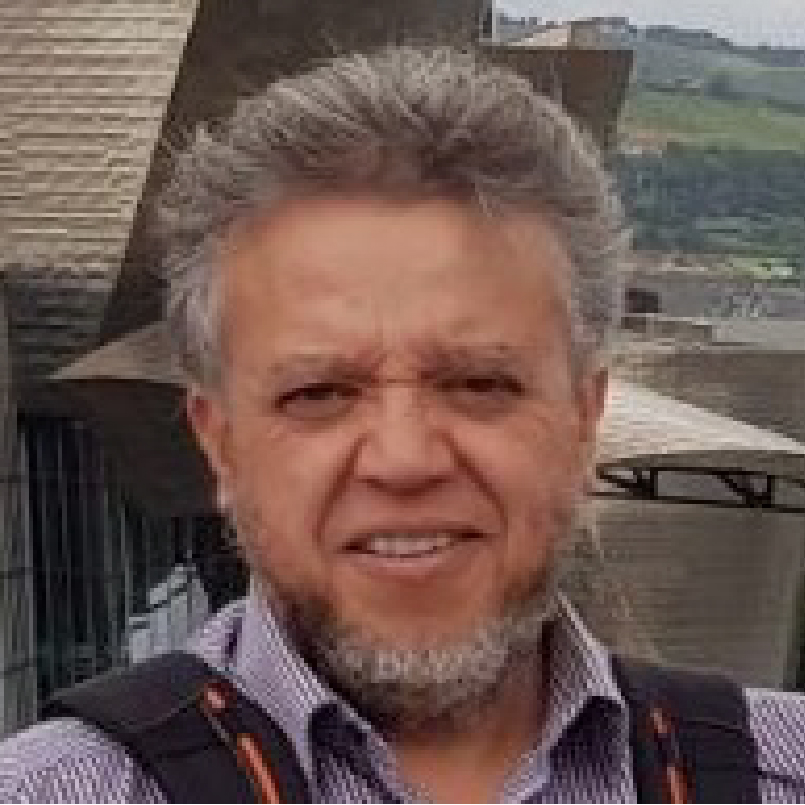
Mohammed Bennamoun
University of Western Australia
3D Computer Vision for Future Robots
Abstract
Robotics has made significant progress in cases of structured and constrained environments, e.g., manufacturing. However, it is still in its infancy when it comes to applications in unstructured and unconstrained situations e.g., social environments. In some respects, such as speed, strength and accuracy, robots have superior capacities compared to humans but that is not the case for person/object recognition, language, manual dexterity, and social interaction and understanding capabilities. Developing a computer vision system with Human visual recognition capabilities has been a very big challenge. It has been hindered mainly by: (i) the non-availability of 3D sensors (with the capabilities of the human eye) which are able to simultaneously capture appearance (colour and texture), surface shapes of objects while in motion, and (ii) the non-availability of algorithms to process this information in real-time. Recently, several affordable 3D sensors appeared in the market which is resulting in the development of practical 3D systems. Examples include 3D object and 3D face recognition for biometric applications, as well as the development of home robotic platforms to assist the elderly with mild cognitive impairment. The objective of the talk will be to describe few 3D computer vision projects and tools used towards the development of a platform for assistive robotics in messy living environments. Various systems with applications and their motivations will be described including 3D object recognition, 3D face/ear biometrics, grasping of unknown objects, and systems to estimate the 3D pose of a person.
Speaker’s Bio
Mohammed Bennamoun is Winthrop Professor in the Department of Computer Science and Software Engineering at the University of Western Australia (UWA) and is a researcher in computer vision, machine/deep learning, robotics, and signal/speech processing. He has published 4 books (available on Amazon), 1 edited book, 1 Encyclopedia article, 14 book chapters, 200+ journal papers, 270+ conference publications, 16 invited and keynote publications. His h-index is 71 and his number of citations is 23,200+ (Google Scholar). He was awarded 70+ competitive research grants, from the Australian Research Council, and numerous other Government, UWA and industry Research Grants. He successfully supervised 30+ PhD students to completion. He won the Best Supervisor of the Year Award at Queensland University of Technology (1998) and received award for research supervision at UWA (2008 and 2016) and Vice-Chancellor Award for mentorship (2016). He delivered conference tutorials at major conferences, including IEEE CVPR 2016, Interspeech 2014, IEEE ICASSP, and ECCV. He was also invited to give a Tutorial at an International Summer School on Deep Learning (DeepLearn 2017).

Enrico Magli
Politecnico di Torino, Italy
Deep learning for inverse problems in imaging
Abstract
Finding solutions to inverse problems has historically been one of the most important image processing problems; deep learning has provided a tremendous boost to the field, allowing to learn complex models that can be used to restore degraded observations. Indeed, recent deep models can be used to learn good image priors in several different ways, leading to plenty of possible applications even with lack of ground truth data, or lack of training data at all!
This talk will focus on recent advances in deep models for restoration, using two application areas as use cases, namely super-resolution and denoising. I will cover models for supervised, self-supervised, unsupervised and one-shot restoration, in the single- and multi-image case, as well as the generation of multiple “good” solutions that are consistent with the observed data. I will show examples of models learning non-local relationships in the data, along with applications to a variety of data types including optical/radar satellite images and point clouds. Finally, I will discuss multimodality as a way to further improve image restoration accuracy.
Speaker’s Bio
Enrico Magli is a Full Professor with Politecnico di Torino, Italy, where he leads the Image Processing and Learning group. He performs research in the fields of deep learning for image and video processing and image compression, with applications to vision and remote sensing. He is a Senior Associate Editor of IEEE Journal on Selected Topics in Signal Processing, and a former Associate Editor of IEEE T-MM and IEEE T-CSVT. He chaired the IEEE Technical Committee on Multimedia Signal processing. He is a Fellow of the IEEE, a Fellow of the ELLIS Society for the advancement of artificial intelligence in Europe, and has been an IEEE Distinguished Lecturer. He was a co-recipient of the IEEE Geoscience and Remote Sensing Society 2011 Transactions Prize Paper Award, the IEEE ICIP 2015 Best Student Paper Award (as senior author), the IEEE ICIP 2019 Best Paper Award, the IEEE Multimedia 2019 Best Paper Award. He has received an ERC consolidator grant in 2011 and an ERC proof-of-concept grant in 2015.
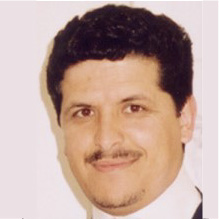
Habib Zaidi
Geneva University Hospital, Switzerland
Quantitative imaging biomarkers
in the era of precision medicine
Abstract
Early diagnosis and therapy increasingly operate at the cellular, molecular or even at the genetic level. As diagnostic techniques transition from the systems to the molecular level, the role of multimodality molecular imaging becomes increasingly important. Positron emission tomography (PET), x-ray computed tomography (CT) and magnetic resonance imaging (MRI) and their combinations (PET/CT and PET/MRI) provide powerful multimodality techniques for in vivo imaging. Quantitative image analysis has deep roots in the usage of molecular imaging in clinical and research settings to address a wide variety of diseases. It has been extensively employed to assess molecular and physiological biomarkers in vivo in healthy and disease states, in oncology, cardiology, neurology, and psychiatry. This talk reflects the tremendous increase in multimodality molecular imaging as both clinical and research imaging modalities in the past decade. An overview of advanced medial image instrumentation technologies and PET image quantification and related image processing issues with special emphasis on radiomics analysis will be presented. This talk aims to bring the biomedical image processing community a review on the state-of-the-art algorithms used and under development for accurate quantitative analysis in multimodality and multiparametric molecular imaging and their validation mainly from the developer’s perspective. It will inform the audience about a series of advanced development recently carried out at the PET instrumentation & Neuroimaging Lab of Geneva University Hospital and other active research groups. Current and prospective future applications of quantitative molecular imaging are also addressed especially its use prior to therapy for dose distribution modeling and optimization of treatment volumes in external radiation therapy and patient-specific 3D dosimetry in targeted therapy towards the concept of image-guided radiation therapy. In this regard, the promising role of artificial intelligence (AI), in particular deep learning approaches, will be emphasized. To this end, example applications of deep learning in five generic fields of multimodality medical image analysis, including imaging instrumentation design, image denoising (low-dose imaging), image reconstruction quantification and segmentation, radiation dosimetry and computer-aided diagnosis and outcome prediction will be discussed. Future opportunities and the challenges facing the adoption of deep learning approaches and their role in molecular imaging research are also addressed.
Speaker’s Bio
Habib Zaidi is Chief physicist and head of the PET Instrumentation & Neuroimaging Laboratory at Geneva University Hospital and full Professor at the medical school of Geneva University. He is also a Professor of Medical Physics at the University of Groningen (Netherlands), Adjunct Professor of Medical Physics and Molecular Imaging at the University of Southern Denmark, Adjunct Professor of Medical Physics at Shahid Beheshti University visiting Professor at Tehran University of Medical Sciences and Distinguished Adjunct Professor at King Abdulaziz University, KSA. He is actively involved in developing imaging solutions for cutting-edge interdisciplinary biomedical research and clinical diagnosis in addition to lecturing undergraduate and postgraduate courses on medical physics and medical imaging. His research is supported by the EEC, Swiss National Foundation, EEC, private foundations and industry (Total 8.8 M US$) and centres on hybrid imaging instrumentation (PET/CT and PET/MRI), deep learning for various imaging applications, modelling medical imaging systems using the Monte Carlo method, development of computational anatomical models and radiation dosimetry, image reconstruction, quantification and kinetic modelling techniques in emission tomography as well as statistical image analysis, and more recently on novel design of dedicated PET and PET/MRI scanners. He was guest editor for 13 special issues of peer-reviewed journals dedicated to Medical Image Segmentation, PET Instrumentation and Novel Quantitative Techniques, Computational Anthropomorphic Anatomical Models, Respiratory and Cardiac Gating in PET Imaging, Evolving medical imaging techniques, Trends in PET quantification (2 parts), PET/MRI Instrumentation and Quantitative Procedures and Clinical Applications, Nuclear Medicine Physics & Instrumentation, and Artificial Intelligence and serves as founding Editor-in-Chief (scientific) of the British Journal of Radiology (BJR)|Open, Deputy Editor for Medical Physics, and Associate Editor or member of the editorial board of the Journal of Nuclear Cardilogy, Physica Medica, International Journal of Imaging Systems and Technology, Clinical and Translational Imaging, American Journal of Nuclear Medicine and Molecular Imaging, Brain Imaging Methods (Frontiers in Neuroscience & Neurology), Cancer Translational Medicine and the IAEA AMPLE Platform in Medical Physics. He has been elevated to the grade of fellow of the IEEE, AIMBE, AAPM, IOMP, AAIA and the BIR and was elected liaison representative of the International Organization for Medical Physics (IOMP) to the World Health Organization (WHO) and Chair of Subcommittee on Part 1 Examination of the International Medical Physics Certification Board (IMPCB) and the Imaging Physics Committee of the AAPM in addition to being affiliated to several International medical physics and nuclear medicine organisations. He is developer of physics webbased instructional modules for the RSNA and Editor of IPEM’s Nuclear Medicine web-based instructional modules. He is involved in the evaluation of research proposals for European and International granting organisations and participates in the organisation of International symposia and conferences. His academic accomplishments in the area of quantitative PET imaging have been well recognized by his peers and by the medical imaging communi ty at large since he is a recipient of many awards and distinctions among which the prestigious 2003 Bruce Hasegawa Young Investigator Medical Imaging Science Award given by the Nuclear Medical and Imaging Sciences Technical Committee of the IEEE, the 2004 Mark Tetalman Memorial Award given by the Society of Nuclear Medicine, the 2007 Young Scientist Prize in Biological Physics given by the International Union of Pure and Applied Physics (IUPAP), the prestigious (100’000$) 2010 kuwait Prize of Applied sciences (known as the Middle Eastern Nobel Prize) given by the Kuwait Foundation for the Advancement of Sciences (KFAS) for “outstanding accomplishments in Biomedical technology”, the 2013 John S. Laughlin Young Scientist Award given by the AAPM, the 2013 Vikram Sarabhai Oration Award given by the Society of Nuclear Medicine, India (SNMI), the 2015 Sir Godfrey Hounsfield Award given by the British Institute of Radiology (BIR), the 2017 IBA-Europhysics Prize given by the European Physical Society (EPS) and the 2019 Khwarizmi International Award given by the Iranian Research Organization for Science and Technology (IROST). Prof. Zaidi has been an invited speaker of over 160 keynote lectures and talks at an International level, has authored over 850+ publications (he is the senior or first author in a majority of these publications), including 375 peer-reviewed journal articles in high ranking journals, most of them in Q1/D1 of their categories (h-index=73, >191’0+ citations | Google scholar), 425 conference proceedings and 42 book chapters and is the editor of four textbooks on Therapeutic Applications of Monte Carlo Calculations in Nuclear Medicine (2 Editions), Quantitative Analysis in Nuclear Medicine Imaging, Molecular Imaging of Small Animals and Computational anatomical animal models.
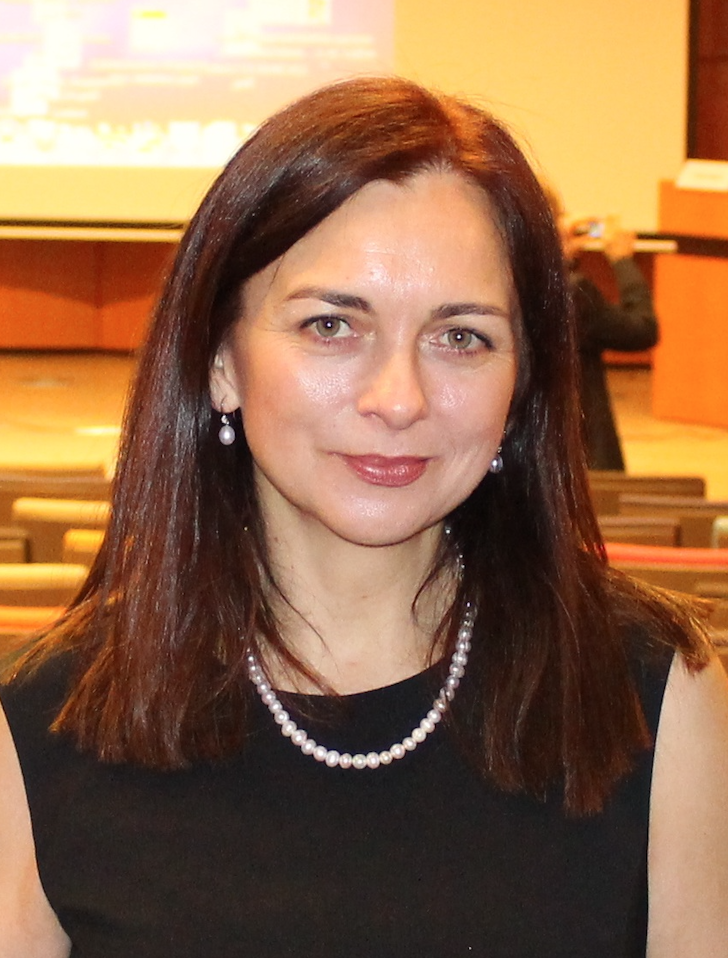
Aleksandra Pizurica
Ghent University, Belgium
Model-Based Optimization Meets Deep Learning in Image Analysis
Abstract
Model-based optimization has been for decades the key to solving not only the classical inverse imaging problems but also various image analysis tasks, such as image classification, clustering, and target detection. We are now experiencing a synergy between deep learning tools and the optimization frameworks benefiting from each other to yield more efficient and reliable inference. Deep sparse representation classification, deep subspace clustering and learned robust PCA are some of the representatives of these emerging approaches.
In this talk, we address model-based approaches for the analysis of hyperspectral and multimodal images. We show how sparse and low-rank optimization methods provide an elegant and unified framework for solving a range of image analysis tasks including classification, clustering, spectral unmixing and saliency detection. Then we discuss model-aware deep learning methods building on this basis. Case studies from different application domains will be presented, including remote sensing and art investigation.
Speaker’s Bio
Aleksandra Pižurica is a Full Professor in Statistical image modelling at Ghent University, Belgium, where she is leading the research group Artificial Intelligence and Sparse Modelling. Her research includes multiresolution image models, probabilistic graphical models, sparse coding, representation learning, and image and video reconstruction, restoration, and analysis. Dr. Pižurica was awarded the scientific prize “de Boelpaepe” by the Royal Academy of Science, Letters and Fine Arts of Belgium for her contributions to statistical image modeling and applications to digital painting analysis, in 2015. She was co-recipient of the Best Paper Award of the IEEE GRSS Data Fusion contest, in 2013 and 2014, and the David Hestenes Prize at AGACSE 2018. She is a Senior Area Editor for the IEEE TIP and formerly served as an Associate Editor of the IEEE TIP and IEEE TCSVT. She was a TPC Co-chair of the 30th EUSIPCO (in 2022), Europe Liaison for ICIP 2020, and the general chair of the Sixth International workshop on Image Processing for Art Investigation (IP4AI) in 2018.